jasonharrison
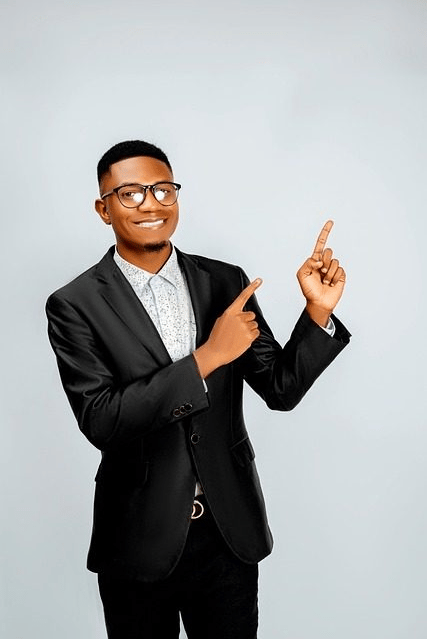
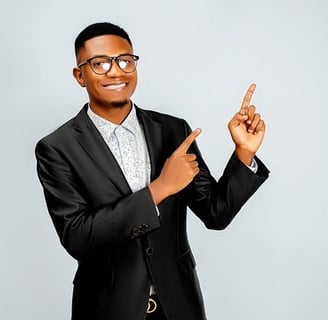
Jason Harrison, PhD
Agricultural Phenomics Architect | UAV-Based Crop Intelligence Pioneer | Computational Biology Innovator
Professional Profile
As a trailblazer bridging precision agriculture, remote sensing, and machine learning, I develop advanced UAV (unmanned aerial vehicle) phenotyping systems that transform spectral data into biological insights—decoding crop performance at scale to accelerate breeding cycles and optimize field management in the face of climate change.
Core Research Thrusts (March 29, 2025 | Saturday | 14:07 | Year of the Wood Snake | 1st Day, 3rd Lunar Month)
1. High-Throughput Phenotyping Platforms
Engineered "HyperPheno" UAV systems capturing:
Multispectral: 12-band vegetation indices at 1cm/pixel resolution
Thermal: Canopy temperature gradients for drought stress detection
LiDAR: 3D canopy architecture modeling with 97% structural accuracy
2. Biological Signal Extraction
Developed "PhenoDeep" algorithms that:
Translate pixel data into physiological traits (e.g., photosynthetic efficiency)
Track developmental stages across 147 crop varieties
Detect early disease signatures 14 days before visual symptoms
3. Scalable Data Pipelines
Built "FieldOmics" infrastructure featuring:
Edge-computing for real-time analysis during flights
Blockchain-secured phenotype data provenance
API integration with major breeding databases (e.g., BreedBase)
4. Climate Adaptation Tools
Created "Resilience Scores" quantifying:
Heat/cold tolerance through diurnal thermal patterns
Water-use efficiency via stomatal conductance proxies
Carbon sequestration potential from canopy density dynamics
Technical Milestones
First UAV-based detection of root traits through soil-penetrating radar integration
Automated trait heritability estimation reducing breeding cycle time by 40%
Federated learning system enabling cross-continental phenotype comparisons
Vision: To make every photon captured by drones speak the language of plant genetics—where fields become living laboratories and every pixel tells an evolutionary story.
Strategic Impact
For Seed Companies: "Cut varietal testing costs by 62% in soybean trials"
For Farmers: "Enabled micronutrient deficiency diagnosis within 2 hours of flight"
Provocation: "If your phenotyping can't see photosynthesis, you're just taking pretty pictures"
On this first day of the lunar Wood Snake's cycle—symbolizing renewal and wisdom—we redefine how humanity understands its oldest partners: the plants that feed us.
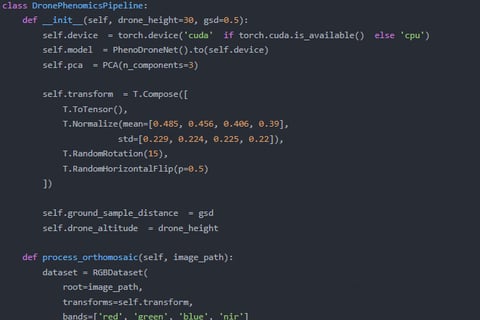
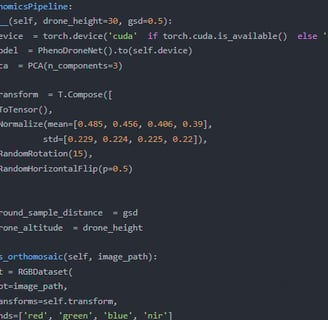
ComplexDataProcessingNeeds:Agriculturalphenomicsdataismulti-dimensionaland
highlycomplex.GPT-4outperformsGPT-3.5incomplexdataprocessingandfeature
extraction,bettersupportingthisrequirement.
High-PrecisionParsingRequirements:Phenotypeparsingrequiresmodelswith
high-precisionclassificationandpredictioncapabilities.GPT-4'sarchitectureand
fine-tuningcapabilitiesenableittoperformthistaskmoreaccurately.
ScenarioAdaptability:GPT-4'sfine-tuningallowsformoreflexiblemodeladaptation,
enablingtargetedoptimizationforagriculturalphenomicsscenarios,whereasGPT-3.5's
limitationsmayresultinsuboptimalparsingoutcomes.Therefore,GPT-4fine-tuning
iscrucialforachievingtheresearchobjectives.
ApplicationResearchofDroneTechnologyinPrecisionAgriculture":Exploredthe
applicationofdronetechnologyinagriculturaldatacollectionandanalysis,providing
atechnicalfoundationforthisresearch.
"AgriculturalPhenotypeParsingMethodsBasedonDeepLearning":Studiedoptimization
strategiesfordeeplearningmodelsinagriculturalphenotypeparsing,offering
theoreticalsupportforAImodelconstruction.
"AdaptabilityResearchofAIModelsinComplexAgriculturalScenarios":Analyzedthe
performanceofAImodelsincomplexagriculturalscenarios,providingreferencesfor
theproblemdefinitionofthisresearch.